
If random events can have a huge impact on health, how can we trust AI predictions? How should leaders navigate the uncertainty of digital transformation?
Interview with Brian Klass, the author of the bestseller Fluke. Chance, Chaos, and Why Everything We Do Matters, and an associate professor in global politics at University College London.
Artur: We are in the middle of the AI revolution. How should we navigate this unpredictable transformative power?
Brian: AI will provide tremendous benefits in a huge array of human realms, but it will be most effective—and safest—in closed systems. For example, AI will be revolutionary in medical diagnoses, as it will be incredibly useful to have models that have been trained on millions of X-rays or MRI scans.
Crucially, though, it’s a closed-system problem—getting it wrong will only affect one individual, not the entirety of society. By contrast, using AI in open systems—such as global economics—will embed catastrophic risk because mistakes can be compounded and global, producing unforeseeable ripple effects.
The challenge is particularly great because AI models are trained on past data. That doesn’t matter in relatively stable realms (trying to diagnose a broken bone is pretty stable over time). But if you’re talking about unstable, ever-changing realms, such as global economics, politics, etc., then AI will provide an illusion of certainty based on past cause-and-effect relationships that may be changing in real time.
Many experts call for AI governance to harness artificial intelligence’s capabilities and mitigate its risks. But can we control how AI will develop by introducing more regulations?
It would be wise to regulate how and when AI systems can be deployed on complex open systems without any human oversight in decision-making. For example, AI, which is used to assist human decision-making, is different from AI, which independently decides.
Take, for example, military targeting. It strikes us as less worrisome to have AI try to identify potential targets that are then confirmed by a human pilot or general compared to an AI system that autonomously decides who to kill and then executes a target independently. The same logic applies to other realms, too. In general, while AI is in its nascent phase, government regulations would be wise to try to involve humans in decision-making that has high-end tail risk (meaning that if it were to go wrong, it could go catastrophically wrong).

“AI models rely on historical data, which works well in stable fields like diagnosing broken bones. However, in dynamic areas such as global economics and politics, AI predictions can be misleading, offering false certainty based on outdated cause-and-effect relationships.”
— Brian Klass
If chance events can change everything, how reliable are the predictions we make in order to co-create the future?
It depends. In certain systems, our predictions can be really, really good.
For example, coin flips offer stable probabilities; we know that, over time, a coin will produce roughly 50% heads and 50% tails. However, trying to accurately predict a single coin flip is impossible.
Similarly, there are some questions for which more data helps you answer the question—such as cancer diagnoses—and some questions for which more data provides no further help. The latter kinds of problems are known as “radical uncertainty,” in which there’s simply no conceivable data that can provide a clear, reliable answer.
The weather, for example, is a chaotic system, meaning that tiny fluctuations can produce enormous changes over time. No matter how powerful our supercomputers become, we will never be able to accurately predict the weather beyond a few weeks. Similarly, if I ask you a question such as “What will the world be like in the year 2050?” there is no data available—or even imaginable—that could guide us to reliably answering that question. It’s the realm of radical uncertainty, and no matter how good our tools get, we’ll never be able to forecast many realms of the world.
What would you advise a leader responsible for digital transformation in an institution? How should strategy be developed when so many things are uncertain?
My grandfather gave me very wise advice. The key to a successful life is simple: avoid catastrophe.
This advice applies to social systems, too. Unfortunately, many of the pressures on leaders are to deliver short-term results even at the cost of significantly amplified long-term risk. I think that’s a mistake. We should be demanding that our leaders think more about avoiding catastrophe and less about squeezing the last three percent of efficiency out of a system, particularly if that extra gain in efficiency comes with the trade-off of reducing slack in the system that could produce greater resilience.
I would much rather live in systems that are stable and avoid catastrophe than ones that might be a bit more efficient now but at the cost of long-term catastrophic risk.
New technologies enable us to control health parameters using wearables to prevent diseases instead of curing them. Do you believe this is possible, considering the theory of chaos? And how can we reduce the impact of things that are out of our control?
Human health is a relatively good system for technology because, in aggregate, the patterns are relatively stable.
We can’t say with 100% certainty that a certain medicine will work or that a certain diet will prevent disease, but we can say with pretty high levels of precision what generally works across populations. That makes many realms of human health more like coin flips – difficult to predict individually but more straightforward to understand when there are thousands or millions of individuals who, taken together, exhibit stable patterns. These same principles should generally apply to most new technologies that aim to tackle problems related to human health.
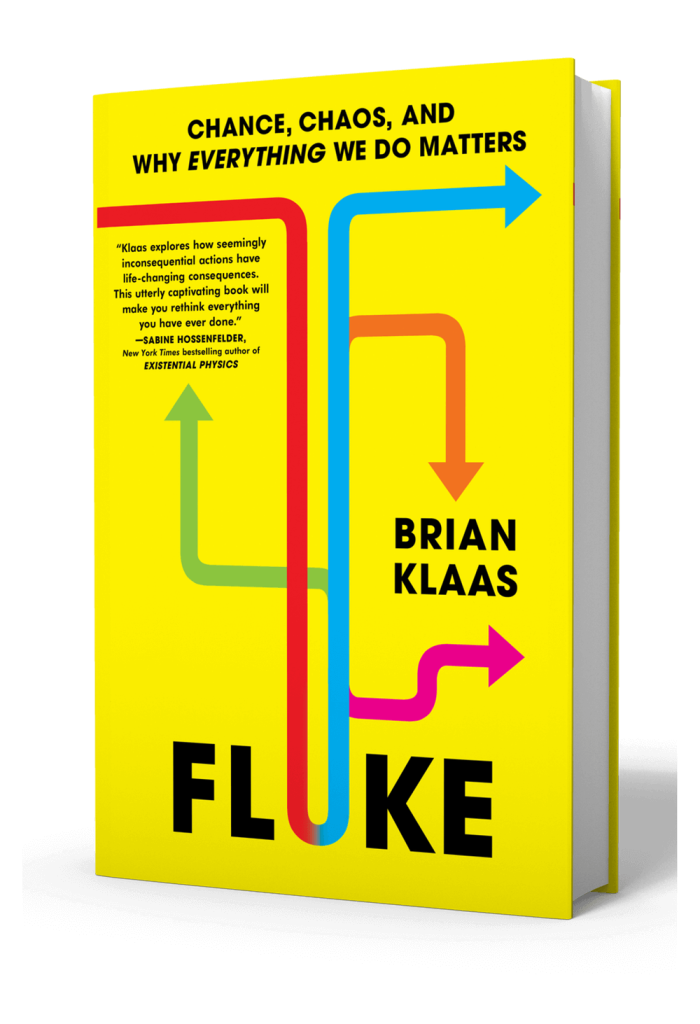
Is data from the past a good predictor of the future?
It depends whether the system exhibits something called non-stationarity or not.
If a system is stationary, it means that cause-and-effect relationships are stable across time and space. For example, mixing baking soda and vinegar produces a fizz. That’s been true forever, and it’s true whether you do it in Malawi or Montana. In that instance, it’s a stationary cause-and-effect dynamic—so the past is an excellent predictor of the future.
However, many complex human systems are not stationary; the underlying dynamics change over time. So, for example, a pandemic is often a non-stationary phenomenon. The 2020 COVID pandemic would have unfolded dramatically differently if it had struck in 1990, not least because few could have worked remotely before the widespread availability of the internet.
Even with the same virus, it would have had different dynamics. Centuries ago, David Hume famously remarked on what he called the problem of induction—that we don’t know that the patterns of the past will predict the future. We can never be certain. But the key is to identify realms that are most stable (more like baking soda and vinegar) because data analytics is most effective in those areas.
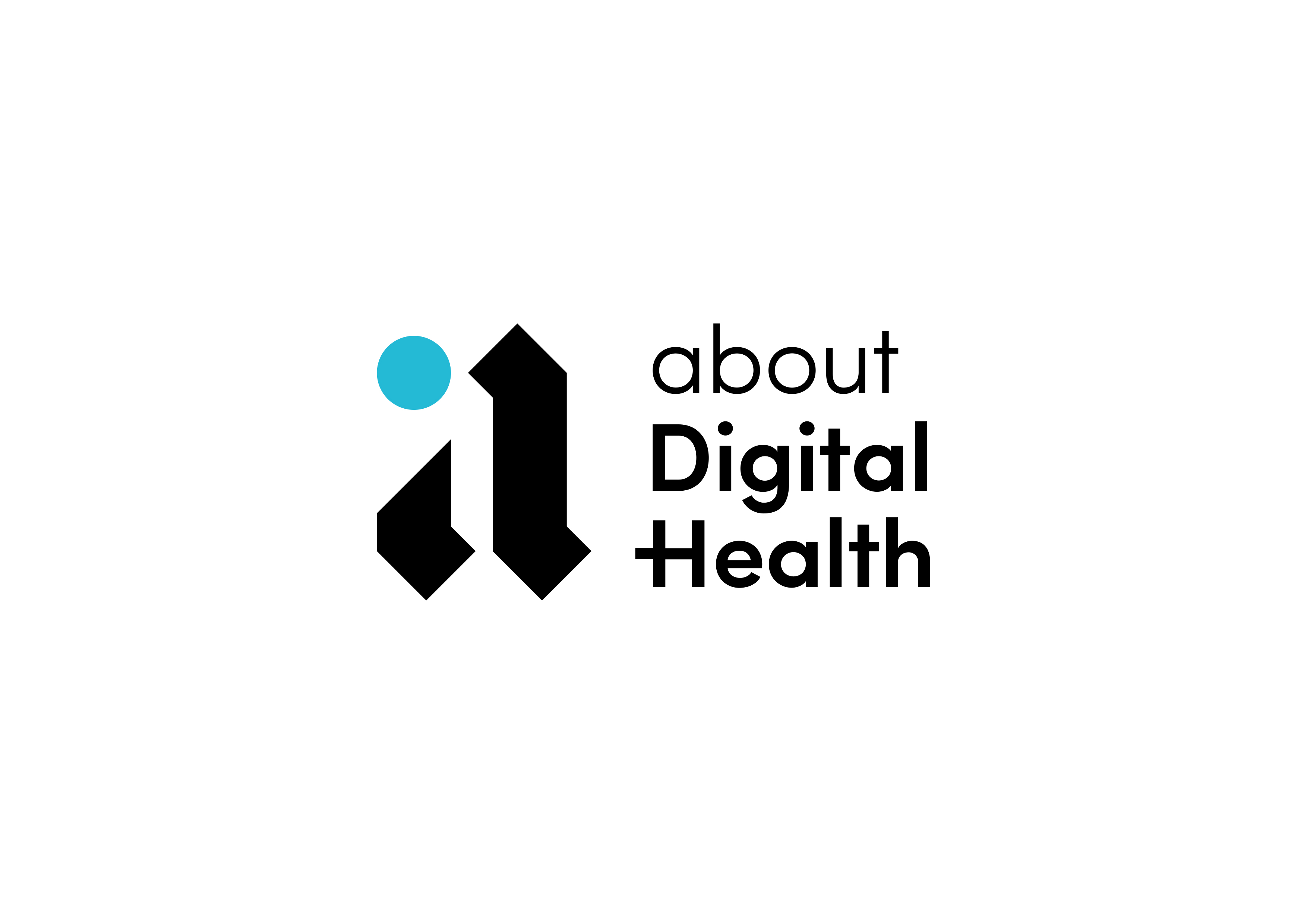
Can I ask you a favor?
Please donate to aboutDigitalHealth to support this not-for-profit knowledge portal. Thank you!
€1.00
One comment